The neglected art of a relevant benchmark and why you need one
Long ago when deep learning was all the rage (circa 2018), you could spend a lot of time and money crunching a lot of data to make a new model and come up with something that was … inconclusively better than what you had had before. Was your model architecture wrong? Could you have picked a better learning rate? Stuck in a local optimum? How to know?
The best way out of the swamp of confusion was to know what you were shooting for. What was a reasonable limit for how good an answer you could get? So we built benchmarks. Unfortunately, a lot of teams working with both traditional and generative AI techniques today aren’t using realistic benchmarks to draw a line in the sand and say ‘we’re aiming for this’.
Let's take a look at why you don't, why you should and how to go about it.
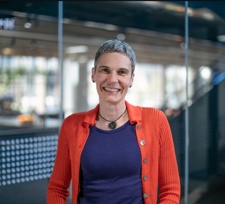
With eight years creating AI-powered SaaS products with global reach, Dr Kendra Vant is an industry leader in harnessing AI and machine learning to solve complex problems with real world impact. She was the Executive GM of Data and AI Product at Xero during the scale up phase, leading the work to help small businesses and their advisors benefit from the power of data and insights. Starting with doctoral research in experimental quantum physics at MIT and a stint building quantum computers at Los Alamos National Laboratory, Kendra has made a career of solving hard problems and pushing the boundaries of what's possible. She currently runs her own consultancy working with executives, boards and founders on practical & ethical applications of AI and is the author of Data Runs Deep, a weekly newsletter exploring the impacts of data and AI in the world today.