ML ain’t your only hammer: adding mathematical optimisation to the data scientist’s toolbox
The purpose of this presentation is to introduce the audience to the field of mathematical optimisation - what it is, how it differs from machine learning, and the types of problems it is best suited to solve.
The first section will provide this context and background - describing the anatomy of an optimisation problem, and what the model building process looks like. We will touch on the characteristics common to optimisation problems seen across multiple industries.
We will then build a simple optimisation model together, demonstrating how the model building process compares to the machine learning approach.
Finally, we will end by exploring some of the most fascinating applications of mathematical optimisation in industry, focussing on the characteristics that the audience can map to challenges that are specific to their own industry.
What do you do if the problem you want to model has little or no clean and available historic data? Or, what if you do have historic data, but things have changed so much that it is no longer possible to use that data to make predictions about the future? On top of that, what if you want your model to incorporate hard constraints that it cannot violate, and these constraints can lead to extremely different modelled outcomes?
Many data scientists would recognise that for these kinds of problems, most machine learning algorithms tend to struggle. However, it is precisely for this reason that the field of mathematical optimisation (MO) was developed. Despite flying relatively under the radar in the data science community, MO plays a critical role solving problems in multiple sectors. The airline industry for example, is so dependent on MO for plane and workforce scheduling, that an entire fleet of planes will be grounded if a bug prevents one of these models solving.
In this presentation, we will introduce the audience to the field of mathematical optimisation, and the types of problems it is exceptionally good at solving. We’ll build a simple model together, and explore how different the approach is to developing a machine learning model. By the end of this talk, we hope many members of the audience will be able to map MO to a problem they are actively trying to solve, and they will be excited to explore applying this technique.
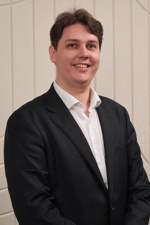
Jack is a Director at Endgame Economics, a boutique consulting firm that specialises in providing quantitative advice in the energy sector. He has over a decade of experience working as a consultant, data scientist, and software developer.
He holds a PhD in computational biology, and has extensive expertise applying mathematical optimisation and machine learning techniques to solve problems in the energy sector.